Like so many other recent graduates I have decided to trade in research in academia for research in the tech industry.
A few years ago, about half way into my PhD program, I wasn't sure what I wanted to do after I graduated. Would I enter the nomadic post-doc life? Am I actually qualified to do anything else? It was at this time I took a class simply called Data Analysis in Astronomy. This class really opened my eyes to a multitude of tools such as: principal component analysis, k-mean clustering, and many other statistical techniques. We had to do a final group project where we developed a facial recognition routine using PCA. This was a fun assignment and really got me thinking about a career where these tools are used in an applied way like this.
The other formative experience was listening to a talk by an astronomy professor/data scientist where he talked about some of the under appreciated results of statistical analysis. For example, he talked about how in Florida right before a hurricane, Targets/Wal-Marts were experiencing a huge spike in sales of a specific item, but it wasn't an obvious one. Not tissue paper, nor bread, nor eggs, nor milk, nor water nor whatever most people would immediately think to stock up on. Instead it was pop-tarts, which kids like, don't need to be heated to eat and are cheap. Here's a perfect example of a result that makes perfect sense when you reflect back on it but wouldn't be immediately apparent upon first thought.
![]() |
(Funny this looks a lot like me! courtesy: http://www.marketingdistillery.com/2014/08/30/data-science-skill-set-explained/) |
After taking that class and listening to that speaker I realized I was more interested in the tools used to analyze data than the data itself. I discovered I wanted to potentially solve a ton more problems than just in astronomy. So I focused my thesis on machine learning (PCA, random forest, time series analysis) so I could more effectively market myself for a post-grad school life.
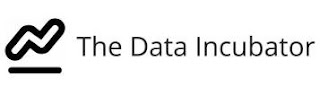
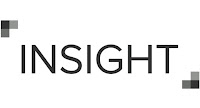
After a short search on Glassdoor, LinkedIn, and other job websites I found my current company, SocialCode. They focus on analyzing ads and ad interactions on social media (e.g. Facebook, Twitter, Instagram).
I applied to a few other places but the interview process was pretty similar for every company. Each began with a very short (~10 min) phone screen just to make sure I was who I said I was. Then a short (~30 min) chat with a current data scientist about my thesis work and they'd ask me some follow-up questions about the data analysis. Sometime in the process, the company would send a short data project that I had 3-7 days to complete. It was usually an open ended question to see how I would analyze data I'd never seen before. This project was then followed by a longer (~45-60 min) chat about the results of the project. Now if they liked what they'd seen and heard from me I'd be invited to an in person interview. At these I would meet with a few current employees and they would grill me on my research, abstract data analysis questions, specific computer science questions among other topics. Honestly, the oral examination of abstract data analysis was much more difficult than defending my thesis!
I'm excited about what the future of data science will bring and how I can contribute, but I'd be lying if I didn't say I was going to miss astronomy. All the wonderful people I've met and interesting projects and teams I've worked on have been a great source of happiness. The academic route was just not for me. Everyone should follow their path as they see it, sometimes that means academia but sometimes not. Don't let anyone else's expectations for you determine your trajectory.
No comments:
Post a Comment